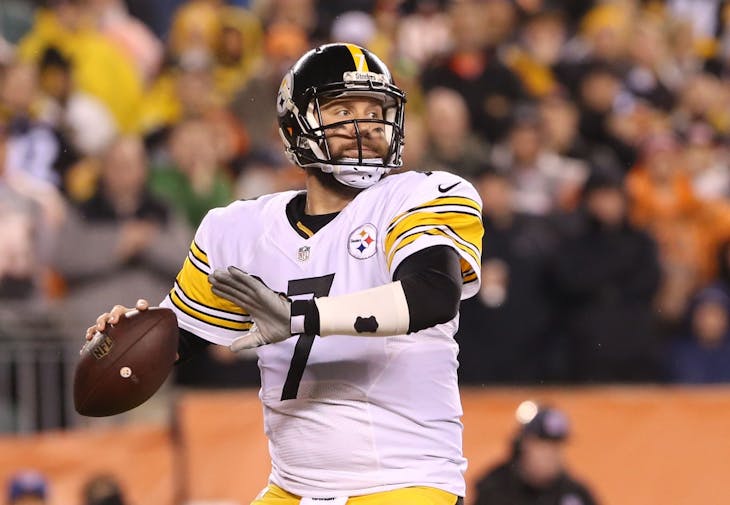
Welcome to Regression Alert, your weekly guide to using regression to predict the future with uncanny accuracy.
The Scorecard
Returning readers, you know how this works by now, but for new readers here's the deal. Every week I take a look at a specific statistic that is prone to regression and identify high and low outliers in that statistic, and then I wave my hands in the air and shout “regression!”
But since predictions aren't any fun without someone holding your feet to the fire afterward, I don't stop there. I lump all of the high outliers into Group A. I lump all of the low outliers into Group B. I verify that Group A is outperforming Group B. And then I predict that Group B will outperform Group A over the next four weeks.
I don't get to pick and choose my groups, beyond being free to pick and choose what statistics are especially prone to regression. If I'm tracking yards per target, and Antonio Brown is one of the high outliers in yards per target, then Antonio Brown goes into Group A and may the fantasy gods show mercy on my predictions.
And then, groups chosen and predictions made, I track my progress. That's this.
In Week 2, I outlined what regression was, what it wasn't, and how it worked. No prediction was made.
In Week 3, I listed running backs with exceptionally high and low yards per carry averages and predicted that the low-ypc cohort would outperform the high-ypc cohort over the next four weeks.
In Week 4, I looked at receivers who were overperforming and underperforming in yards per target and predicted that the underperformers would outperform the overperformers over the next four weeks.
In Week 5, I compared the predictive accuracy of in-season results to the predictive accuracy of preseason ADP. Outside of a general prediction that players would tend to regress in the direction of their preseason ADP, no specific prediction was made.
In Week 6, I looked at quarterbacks who were throwing too many or too few touchdowns given the amount of passing yards they were accumulating, then predicted that the underperformers would score more fantasy points than the overperformers going forward.
In Week 7, I looked at receivers who were catching too many or too few touchdowns based on their yardage total, then predicted that the underperformers would score more fantasy points than the overperformers going forward.
In Week 8, I revisited yards per carry, again predicting that the high-carry, low-ypc group would outrush the low-carry, high-ypc group going forward.
In Week 9, I went back to yard to touchdown ratios, predicting that the low-touchdown group would close the gap substantially with the high-touchdown group going forward.
In Week 10, I discussed the pitfalls of predicting regression over 4-week windows. No specific prediction was made.
In Week 11, I once more delved into the theory behind regression and highlighted the importance of not cherrypicking which players are “too good” or “not good enough” to regress.
In Week 12, I took one more shot at touchdown regression for quarterbacks, predicting that the low-touchdown cohort would close the gap with the high-touchdown cohort going forward.
In Week 13, I decided to close the predictions out with a bang, sorting the top 100 skill position players in yards per touchdown ratio and predicting that the third with the fewest touchdowns would outperform the third with the most touchdowns going forward.
In Week 14, I delved into Alvin Kamara's aberrant touchdown rate, whether sometimes outliers were actually indicators, and whether anyone could possibly be “regression-proof”.
In Week 15, we took a break before the end-of-season wrapup articles this week and next.
In Week 16, I did a deep dive on quarterback yard-to-touchdown ratios to see whether this year's poor performance was an outlier.
Statistic for regression | Performance before prediction | Performance since prediction | Weeks remaining |
---|---|---|---|
yards per carry | Group A had 60% more rushing yards per game | Group B has 16% more rushing yards per game | None (Win!) |
yards per target | Group A had 16% more receiving yards per game | Group B has 11% more receiving yards per game | None (Win!) |
passing yards per touchdown | Group A had 13% more fantasy points per game | Group A has 17% more fantasy points per game | None (Loss) |
receiving yards per touchdown | Group A had 28% more fantasy points per game | Group B has 1% more fantasy points per game | None (Win!) |
yards per carry | Group A had 25% more fantasy points per game | Group B has 16% more fantasy points per game | None (Win!) |
rushing yards per touchdown | Group A had 21% more fantasy points per game | Group B has 8% more fantasy points per game | None (Win!) |
passing yards per touchdown | Group A had 14% more fantasy points per game | Group A has 23% more fantasy points per game | None (Loss) |
yards from scrimmage per touchdown | Group A had 7% more fantasy points per game | Group B has 20% more fantasy points per game | None (Win!) |
Regression to the mean closes out our final prediction of the season in decisive fashion. Remember, heading into week 13, Group A had outscored Group B by 7% on the season. In the four weeks since, Group A's best week produced an 8.28 point average, while Group B's worst week produced an 8.45 point average.
The touchdown-dependent players in Group A scored fewer than 5 points 41.4% of the time, vs. just 33.6% of games over 10 points. In yardage-dependent Group B, only 31.0% of games were below five points, while 37.7% scored 10 points or more. Even with three-quarters of a season worth of data to establish our groups, regression still hit quickly and hit hard.
Season-long wrap-up
If there's one point I've tried to stress over and over again this year, it's that regression operates over larger timescales. The “test each prediction after four weeks” model is fantastic for accountability, but it gives us to a limited picture of regression's true power.
To that end, I wanted to revisit all of my predictions from the first half of the season and fill in data from the entire year instead of just the four weeks that immediately followed.
Statistic for regression | Performance before prediction | Performance in the 4-week span | Performance over the entire remaining season |
---|---|---|---|
yards per carry | Group A had 60% more rushing yards per game | Group B had 16% more rushing yards per game | Group B had 28% more rushing yards per game |
yards per target | Group A had 16% more receiving yards per game | Group B had 11% more receiving yards per game | Group B had 11% more receiving yards per game |
passing yards per touchdown | Group A had 13% more fantasy points per game | Group A had 17% more fantasy points per game | Group A had 2% more fantasy points per game |
receiving yards per touchdown | Group A had 28% more fantasy points per game | Group B had 1% more fantasy points per game | Group B had 11% more fantasy points per game |
yards per carry | Group A had 25% more fantasy points per game | Group B had 16% more fantasy points per game | Group B had 19% more fantasy points per game |
rushing yards per touchdown | Group A had 21% more fantasy points per game | Group B has 8% more fantasy points per game | Group A had 1% more fantasy points per game |
Yards per Carry: The most dramatic part of this prediction is not just that Group B managed to widen its lead over the remainder of the season. It's that Group B actually averaged more yards per carry than Group A over the remainder of the season.
Remember, every single running back in Group A averaged 4.4 yards per carry or better at the time of the prediction, and the group as a whole averaged 5.70 yards per carry. Meanwhile, every single running back in Group B averaged 3.5 yards per carry or worse, and the group as a whole averaged just 3.16 yards per carry.
From week three through today, Group A averaged 3.97 yards per carry, while Group B averaged 4.07 yards per carry. The average gain for all running back carries in the entire NFL this season was 4.03. So the very best in yards per carry and the very worst in yards per carry were basically average going forward.
Yards per carry is basically meaningless.
Yards per Target: Unlike yards per carry, the best receivers in yards per target actually managed to maintain their lead over the worst receivers in yards per target. But the low-YPT receivers' volume advantage proved decisive.
Prior to the prediction, Group A averaged 11.7 yards per target. After the prediction, that fell all the way to 8.2 yards per target. Group B, on the other hand, saw its yards per target average rise from 7.1 before the prediction to 7.8 after. Instead of Group A gaining an extra 4.6 yards for every target, they only gained 0.4 yards per target; with Group B getting more than one extra target per game, there was no way for Group A to keep pace.
Across all wide receivers, the average yards per target this year was 7.6, so both Group A and Group B were above-average, (which is unsurprising because we were only looking at productive fantasy receivers, who are almost by definition above-average players).
Passing Yards per Touchdown: This one might be a huge surprise given how poorly predictions of quarterback regression fared in-sample this season, but given another seven weeks, Group B actually almost managed to pass Group A after all!
From the time of the prediction to today, quarterbacks in Group A, (those who were throwing more touchdowns than we should expect), saw their average points per game fall by 2.5 points. Quarterbacks in Group B, (those who were throwing too few touchdowns), saw their average fall by just 0.3 points per game.
Three quarterbacks— Deshaun Watson and Aaron Rodgers in Group A, Carson Palmer in Group B— played just two games after the prediction, which means they didn't have an opportunity for regression to act over a longer timescale. If we remove those three quarterbacks from the sample, then Group A averaged 19.90 points per game since the prediction, while Group B averaged... 19.90 points per game since the prediction.
Alternately, if we just look at how these groups have fared since the 4-week window ended, Group B averaged 20.33 points per game while Group A averaged 18.85 points per game, an 8% advantage for Group B.
It seems quarterbacks weren't immune to regression this season, after all, I just picked a terrible window to track them during.
Receiving Yards per Touchdown: At the time of the prediction, Group A averaged 53.4 yards and 0.77 touchdowns per game. Since the prediction, Group A averaged 59.4 yards and 0.40 touchdowns per game.
At the time of the prediction, Group B averaged 71.7 yards and 0.10 touchdowns per game. Since the prediction, Group B averaged 67.6 yards and 0.41 touchdowns per game. Yes, Group B— the “low touchdown” group— scored more touchdowns than Group A— the “high touchdown” group.
Group A saw its yardage average increase by 11%. Group B saw its yardage average decrease by 6%. Yardage, it turns out, is a very stable predictor. Group A saw its touchdowns decrease by 48%. Group B saw its touchdowns increase by 410%. Touchdowns, it turns out, are completely random and unpredictable.
When given the opportunity to bet on a guy with a lot of yards or a guy with a lot of touchdowns, bet on the guy with the yards every time.
Yards per Carry Redux:
Group A: 3.94 yards per carry since the prediction
Group B: 4.22 yards per carry since the prediction
Say it with me now: yards per carry is basically meaningless.
Rushing yards per Touchdown:
Group A: 0.62 touchdowns per game since the prediction
Group B: 0.62 touchdowns per game since the prediction
Again, the fact that Group A had a ton of touchdowns held basically no predictive power, because touchdowns hold basically no predictive power.
So why has Group A managed to overtake Group B since the prediction again? This is where small sample sizes can create odd artifacts in the data. Since the prediction, Group A could have played in 39 possible games. Instead, they combined to miss 13 of those games, (five by Chris Thompson, six by Ezekiel Elliott, and two by Leonard Fournette). Group B, on the other hand, didn't miss a single game.
The result is that the two Group A backs who stayed healthy, (Todd Gurley and Melvin Gordon), saw their production receive extra weight in the final average. Todd Gurley alone accounted for 30% of the games played by Group A, and Todd Gurley was a monster down the stretch. Group A was essentially rewarded for the fact that most of its backs couldn't stay on the field.
Meanwhile, the best Group B back, Le'Veon Bell, only accounted for 19% of the total games played by Group A. If Bell was weighted so he accounted for 30% of his group's production like Gurley did, Group B would have outperformed Group A by 3%. If Gurley was weighted so he accounted for 19% of Group A's production like Bell did, Group B would have outperformed Group A by 8%. If you just take a simple average of each player's point per game average, Group B outperformed Group A by 10%.
And of course, all of this is a great illustration of why large sample sizes are such an asset for regression to the mean.
Anyway, thanks to everyone who followed along this season. Hopefully, I've managed to demonstrate just how powerful regression to the mean really is, how it's able to turn average fans into prescient prognosticators with minimal knowledge and effort.