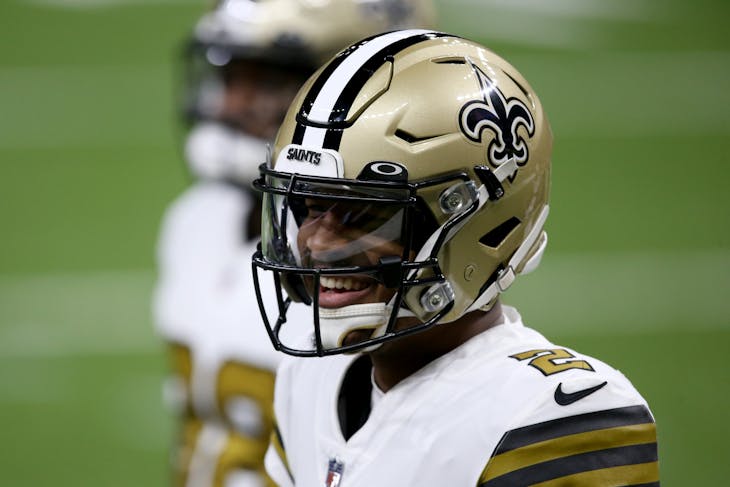
Welcome to Regression Alert, your weekly guide to using regression to predict the future with uncanny accuracy.
For those who are new to the feature, here's the deal: every week, I dive into the topic of regression to the mean. Sometimes, I'll explain what it really is, why you hear so much about it, and how you can harness its power for yourself. Sometimes, I'll give some practical examples of regression at work.
In weeks where I'm giving practical examples, I will select a metric to focus on. I'll rank all players in the league according to that metric and separate the top players into Group A and the bottom players into Group B. I will verify that the players in Group A have outscored the players in Group B to that point in the season. And then I will predict that, by the magic of regression, Group B will outscore Group A going forward.
Crucially, I don't get to pick my samples (other than choosing which metric to focus on). If I'm looking at receivers and Justin Jefferson is one of the top performers in my sample, then Justin Jefferson goes into Group A, and may the fantasy gods show mercy on my predictions.
Most importantly, because predictions mean nothing without accountability, I report on all my results in real time and end each season with a summary. Here's a recap from last year detailing every prediction I made in 2022, along with all results from this column's six-year history (my predictions have gone 36-10, a 78% success rate). And here are similar roundups from 2021, 2020, 2019, 2018, and 2017.
The Scorecard
In Week 2, I broke down what regression to the mean really is, what causes it, how we can benefit from it, and what the guiding philosophy of this column would be. No specific prediction was made.
In Week 3, I dove into the reasons why yards per carry is almost entirely noise, shared some research to that effect, and predicted that the sample of backs with lots of carries but a poor per-carry average would outrush the sample with fewer carries but more yards per carry.
In Week 4, I explained that touchdowns follow yards, but yards don't follow touchdowns, and predicted that high-yardage, low-touchdown receivers were going to start scoring a lot more going forward.
In Week 5, we revisited one of my favorite findings. We know that early-season overperformers and early-season underperformers tend to regress, but every year, I test the data and confirm that preseason ADP is still as predictive as early-season results even through four weeks of the season. I sliced the sample in several new ways to see if we could find some split where early-season performance was more predictive than ADP, but I failed in all instances.
In Week 6, I talked about how when we're confronted with an unfamiliar statistic, checking the leaderboard can be a quick and easy way to guess how prone that statistic will be to regression.
STATISTIC FOR REGRESSION | PERFORMANCE BEFORE PREDICTION | PERFORMANCE SINCE PREDICTION | WEEKS REMAINING |
---|---|---|---|
Yards per Carry | Group A had 42% more rushing yards per game | Group A has 10% more rushing yards per game | None (Loss) |
Yard-to-TD Ratio | Group A had 7% more points per game | Group B has 38% more points per game | None (Win) |
Passing Yards | Teams averaged 218.4 yards per game | Teams average 221.3 yards per game | 10 |
Our high-touchdown receivers finally came alive in the last week of the prediction, scoring seven touchdowns in ten games, but it was too little, too late. The low-yardage receivers remained low-yardage (going from 57.8 yards per game to 53.0 yards per game), the high-yardage receivers continued getting lots of yards (going from 85.3 to 77.2 yards per game), and while Group A continued doing a better job converting yards into touchdowns (scoring once for every 136 yards compared to once per 168 yards for Group B), the fact that Group B had more yards to convert means they also scored more touchdowns overall (0.46 per game compared to 0.39 per game).
Overall, Group A underperformed their initial average in three out of four weeks since our prediction, while Group B overperformed their starting average in all four weeks.
Our passing yards per game prop had a poor first week as teams averaged 240 yards per game, but a lot of the value proposition here was that passing would decline as the weather turned bad, so there's still a long way to go.
(Most) Quarterback Stats Don't Regress As Much
When explaining how regression works, I mentioned that all production is partly a result of skill (or factors innate to the player) and partly a result of luck (or factors outside the player's direct control). Statistics that are more luck than skill tend to regress quicker and more significantly than statistics that are more skill than luck.
I linked to research by Danny Tuccitto, finding that running backs needed 1,978 carries before their ypc average reached a point where it represented more skill than luck. Using the same methodology, Danny found that a quarterback's yards per attempt average (or YPA) stabilized in just 396 attempts. For a running back, that represents eight years of 250-carry seasons. For a quarterback, that's less than a season's worth of attempts (only one team -- last year's Chicago Bears -- has finished with fewer than 400 pass attempts in the last two seasons).
As a result, you're not going to see me predicting regression very often for quarterback stats like yards per attempt. (In fact, the last time I did so was in 2020 when I predicted-- successfully -- that yards per attempt wouldn't regress.)
But there is one quarterback statistic that I love to badmouth, one that is terrible, horrible, no good, very bad. That statistic is interception rate.
Continue reading this content with a PRO subscription.
"Footballguys is the best premium
fantasy football
only site on the planet."
Matthew Berry, NBC Sports EDGE