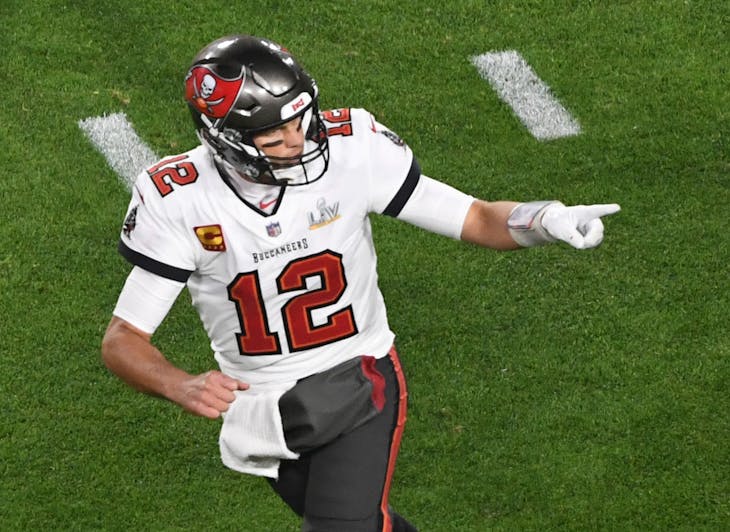
Welcome to Regression Alert, your weekly guide to using regression to predict the future with uncanny accuracy.
For those who are new to the feature, here's the deal: every week, I dive into the topic of regression to the mean. Sometimes, I'll explain what it really is, why you hear so much about it, and how you can harness its power for yourself. Sometimes, I'll give some practical examples of regression at work.
In weeks where I'm giving practical examples, I will select a metric to focus on. I'll rank all players in the league according to that metric and separate the top players into Group A and the bottom players into Group B. I will verify that the players in Group A have outscored the players in Group B to that point in the season. And then I will predict that, by the magic of regression, Group B will outscore Group A going forward.
Crucially, I don't get to pick my samples (other than choosing which metric to focus on). If I'm looking at receivers and Justin Jefferson is one of the top performers in my sample, then Justin Jefferson goes into Group A, and may the fantasy gods show mercy on my predictions.
Most importantly, because predictions mean nothing without accountability, I report on all my results in real time and end each season with a summary. Here's a recap from last year detailing every prediction I made in 2022, along with all results from this column's six-year history (my predictions have gone 36-10, a 78% success rate). And here are similar roundups from 2021, 2020, 2019, 2018, and 2017.
The Scorecard
In Week 2, I broke down what regression to the mean really is, what causes it, how we can benefit from it, and what the guiding philosophy of this column would be. No specific prediction was made.
In Week 3, I dove into the reasons why yards per carry is almost entirely noise, shared some research to that effect, and predicted that the sample of backs with lots of carries but a poor per-carry average would outrush the sample with fewer carries but more yards per carry.
In Week 4, I explained that touchdowns follow yards, but yards don't follow touchdowns, and predicted that high-yardage, low-touchdown receivers were going to start scoring a lot more going forward.
In Week 5, we revisited one of my favorite findings. We know that early-season overperformers and early-season underperformers tend to regress, but every year, I test the data and confirm that preseason ADP is still as predictive as early-season results even through four weeks of the season. I sliced the sample in several new ways to see if we could find some split where early-season performance was more predictive than ADP, but I failed in all instances.
In Week 6, I talked about how when we're confronted with an unfamiliar statistic, checking the leaderboard can be a quick and easy way to guess how prone that statistic will be to regression.
STATISTIC FOR REGRESSION | PERFORMANCE BEFORE PREDICTION | PERFORMANCE SINCE PREDICTION | WEEKS REMAINING |
---|---|---|---|
Yards per Carry | Group A had 42% more rushing yards per game | Group A has 10% more rushing yards per game | None (Loss) |
Yard-to-TD Ratio | Group A had 7% more points per game | Group B has 48% more points per game | 1 |
It was looking for a moment like we might manage to salvage our yards per carry prediction. Group A had its worst week of the sample, averaging just 50 rushing yards on just 3.85 yards per carry. If Group B could simply maintain their average over the last three weeks (60.5 yards, 3.93 yards per carry) they would have completed an unlikely come-from-behind victory. Unfortunately, Group B also had their worst week of the season so far (42.2 yards, 3.72 yards per carry), and our perfect record on this prediction has finally come to an end.
I've always said that the streak would end eventually, and looking back, the things that brought it down aren't the least bit surprising. If yards per carry from one sample to the next is really random, eventually we'd expect the high-YPC group to maintain a high ypc and the low-YPC group to maintain a low ypc just by chance alone, and that's what we saw (4.66 ypc for Group A, 3.89 ypc for Group B).
Despite that, we still saw a 32% swing from Group A toward Group B, which is close to the median value we see on this prediction (39%), but there we bump into the second problem-- 43% is the second-largest lead Group A has had over Group B in our ten attempts at this prediction. Given a more typical edge (20-25%), a 32% swing would have been enough to flip the results. But a larger-than-typical starting gap paired with a smaller-than-typical yards per carry regression means we come up a hair short. All good things come to an end, but we'll revisit this later in the season and see if we can start a new winning streak.
At least our Yards Per Touchdown prediction keeps chugging along. After scoring a ridiculous 14 touchdowns in the first two weeks, our "low-touchdown" receivers were shut out of the end zone in Week 6... but outscored Group A on the week anyway, thanks to an 85.0 to 51.9 yard per game advantage.
At the time of the prediction Group A averaged 57.8 yards per game and Group B averaged 85.3. Since the prediction, Group A averages 53.0 yards per game and Group B averages 75.5. Yardage, as you can see, is fairly stable across samples. Touchdowns? Not so much. Group A has gone from one touchdown for every 75.6 yards to one touchdown for every 182.6 yards. Group B has gone from one touchdown for every 469 yards to one touchdown for every 161.7 yards. So not only is Group B gaining more yards, but they're also converting those yards into touchdowns at a slightly better rate (though both groups' averages are within the "sustainable band" I mentioned at the outset).
Must Historical Outliers Regress?
Last week, I wrote about how, when confronted with an unfamiliar statistic, we can often use our intuitions to make a fairly accurate guess of how much it will regress. This time last year, I followed that lesson up with a practical example, writing about how NFL games were closer than at nearly any point in history (as measured by margin of victory, at the time just 9.05 points per game). I then discussed how I had no idea what a "normal" margin of victory "should" look like and walked through the process of taking a completely new statistic and making estimates about how it was going to behave going forward. (I predicted margin of victory would settle between 9.0 and 10.5 over the ensuing four weeks, which was substantially below the 2021 average of 12.2 points per game. It wound up being 9.9.)
That was a fun experiment, so when I saw another example from the "This Season's Statistics Are Wildly Out Of Line With Recent Historical Averages" genre, I figured we could run it back.
Average Passing Yards Per Team, Per Game, By Year
— Russell Clay (@RussellJClay) October 18, 2023
(Through Week 6 of each year)
2018: 272.6
2019: 258.5
2020: 259.2
2021: 263.4
2022: 240.9
2023: 236.3
Hard to believe these are even real numbers.
This is very cool! (My idea of "cool" might differ slightly from yours.) Unlike last year's example, I've spent quite a lot of time looking at historical offensive trends, so this one doesn't come as a surprise to me. I knew passing yards per game was down significantly last year, and it certainly felt like it had gotten even lower this year. But is this a sign of things to come or just a 6-week fluke?