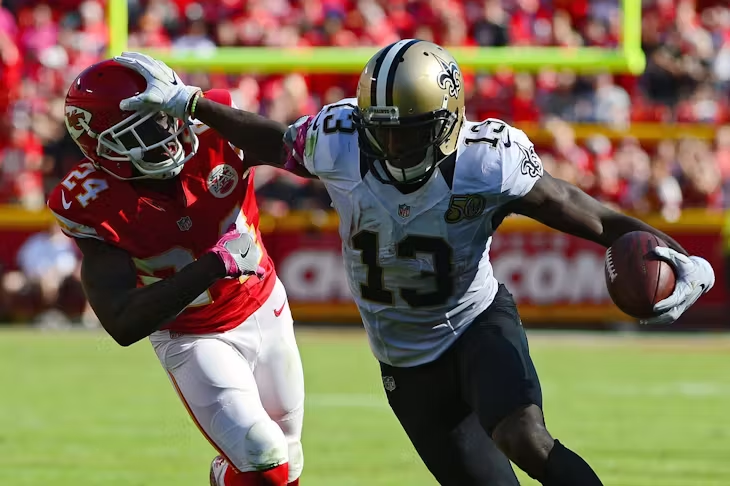
Welcome to Regression Alert, your weekly guide to using regression to predict the future with uncanny accuracy.
For those who are new to the feature, here's the deal: every week, I dive into the topic of regression to the mean. Sometimes I'll explain what it really is, why you hear so much about it, and how you can harness its power for yourself. Sometimes I'll give some practical examples of regression at work.
In weeks where I'm giving practical examples, I will select a metric to focus on. I'll rank all players in the league according to that metric and separate the top players into Group A and the bottom players into Group B. I will verify that the players in Group A have outscored the players in Group B to that point in the season. And then I will predict that, by the magic of regression, Group B will outscore Group A going forward.
Crucially, I don't get to pick my samples (other than choosing which metric to focus on). If I'm looking at receivers and Cooper Kupp is one of the top performers in my sample, then Cooper Kupp goes into Group A and may the fantasy gods show mercy on my predictions.
Most importantly, because predictions mean nothing without accountability, I track the results of my predictions over the course of the season and highlight when they prove correct and also when they prove incorrect. At the end of last season, I provided a recap of the first half-decade of Regression Alert's predictions. The executive summary is we have a 32-7 lifetime record, which is an 82% success rate.
If you want even more details here's a list of my predictions from 2020 and their final results. Here's the same list from 2019 and their final results, here's the list from 2018, and here's the list from 2017.
What Is Regression To The Mean
For our first article of the year, I think it's important to nail down exactly what regression to the mean is and why it is so powerful. I'd like to illustrate it with an example from basketball.
The free throw attempt might be the purest act in all of sports. There's no defense. There's no weather. The distance and angle never change. It is exactly the same every time: one player, one ball, one hoop, one shot.
For his career, Steph Curry shoots 90% on free throws, but on a game-to-game level, there's a little bit of variance. Imagine that in Week 1 of the 2022-2023 season, Curry makes 3 of 6 free throws. (This would be a wildly uncharacteristic game, but it's not impossible; Curry once shot 1-of-4 and has twice gone 4-of-7.)
Nobody in their right mind would look at this game and conclude that Curry was suddenly a 50% free throw shooter, right? Instead, we'd think this game was an outlier and expect him to go back to hitting 90% the rest of the way. Because 90% is Curry's long-term mean (or average), and we expect him to regress (or return) to it.
Just like Steph Curry has an innate average free throw percentage, so does every player have an innate average talent level. And just as Curry's game-by-game results can deviate from that average, so can every player's results deviate from their own true mean. And just like we'd expect Curry to return to his average, we should expect all players to return to theirs, as well.
That's regression to the mean in a nutshell. It's a concept we all intuitively understand, even if we don't talk about it in so many words.
And if we're going to take advantage of regression to the mean, there are four guiding principles we need to keep in mind.