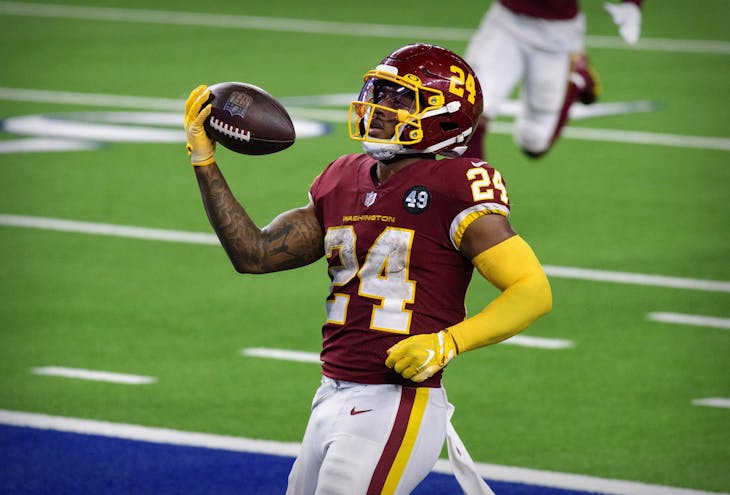
There's a lot of really strong dynasty analysis out there, especially when compared to five or ten years ago. But most of it is so dang practical-- Player X is undervalued, Player Y's workload is troubling, the market at this position is irrational, take this specific action to win your league. Dynasty, in Theory is meant as a corrective, offering insights and takeaways into the strategic and structural nature of the game that might not lead to an immediate benefit, but which should help us become better players over time. (Additionally, it serves as a vehicle for me to make jokes like "theoretically, this column will help you out".)
How to be Wrong
In my two decades of playing, writing, and thinking about fantasy football, I don't know if there's a more important lesson I've learned than "how to be wrong". By this, I don't mean "what one must do in order to be wrong"-- it certainly didn't take me 20 years to figure that one out, and you can certainly manage that well enough without my help. Even the best of us certainly believes more wrong things than right things at any given moment.
No, the goal is to be right, and to accomplish that goal, we must consistently discard all those wrong beliefs so we can replace them with marginally-less-wrong ones. And the only way to accomplish that is to acknowledge that those wrong beliefs are, in fact, wrong. "Being wrong" is a crucial step on the path to "being right", and the managers who can accomplish that the quickest and most consistently are the ones who will have the highest percentage of surviving beliefs that are actually correct.
Austin Ekeler was an undrafted rookie who wasn't expected to make the final 53-man roster, let alone go on to become a fantasy contributor, let alone go on to become one of the most valuable running backs in the league. Nobody thought he would become what he has become, which means everybody was wrong about him. And the managers who recognized that error first were the managers who benefited from that error most.
So the question becomes "how can we be wrong as quickly and efficiently as possible (so that we can start being right)". The most trivial way is for an outside party to present us with compelling enough evidence to get us to change our minds. In the case of Ekeler, the man himself disabused us of any incorrect notions; it's hard to believe he won't be fantasy-relevant in the face of a Top-30 finish as a sophomore and harder still when confronted by a Top-5 finish in Year 3.
Occasionally, a third party can present a compelling enough argument to cause us to update our beliefs. Matt Waldman got his start in the fantasy football industry by inventing "Crank Scores", which measured the consistency of a player's weekly production (Crank = C-Rank = Consistency Rank). Another analyst presented a strong argument that consistency was not a meaningful lens through which to view player production and (after stewing on it for a bit), Waldman abandoned his work on the subject despite it having received plenty of positive traction overall. (A willingness to be argued out of a popular and productive stance is extraordinarily rare; Matt Waldman is one of the best analysts in football at being wrong, which is why he's one of the best analysts in football.)
But we can't always rely on strangers to find our wrong beliefs for us. Nor can we just discard our beliefs every time a stranger tells us we're wrong. (After all, on average strangers are just as likely to be wrong as we are.) Science grappled with this problem and came up with the scientific method, including the key precept of falsifiability. To justify believing something, scientists must first try their hardest to prove it wrong.
Science isn't perfect, but it has a pretty impressive track record. It is how, in the span of a single human lifetime, we progressed from first sketching out a plan to send objects up into space to successfully launching them at asteroids with enough force to alter their trajectory.
So, in the spirit of science and to provide an example of how to be wrong, I wanted to subject one of my most profitable beliefs to rigorous scrutiny.
Revisiting "Revisiting Preseason Expectations"
In my first year writing for Footballguys, I investigated after Week 4 how much predictive weight preseason ADP still carried. It's one of my favorite investigations because it found that preseason ADP was still as predictive as results to date. I liked it so much that I repeated the analysis after Week 4 again the next year, and the year after that, and the year after that, and so on. Last week I completed the tenth annual investigation of the predictive power of ADP. This has been a very beneficial series for me; it is constantly referenced in the industry and is one of the things I have come to be best known for.
I'm also not entirely sure if it's right.
There are several problems with the methodology. Discarding players who miss too much time reduces the signal (and will fail to catch players who miss time because they got benched for not playing well). Looking at positional rank instead of production exaggerates the sizes of some gaps while minimizing the size of others (there might be a 3-point per game difference between 1st and 2nd but only a 2-point per game difference between 32nd and 64th).
So why did I use this methodology in the first place? Because I was a less experienced analyst and because I had much weaker data sources available to me at the time. And why have I stuck with the same methodology over the years? Because I want current results to be directly comparable to past results.
And also because the current methodology keeps proving me right and I have a pretty vested interest in appearing right on this subject. But at the end of the day, it's more important to be right than to merely appear right, so let's use a better methodology and subject this belief to critical examination.
Defining The Methodology
The first step in falsifying a belief is declaring in advance what it will take to change your mind. If you look at the evidence first it's easy to find justifications, so it's best to commit in advance. I want to pay special attention to the potential failure modes of the old methodology.
I mentioned several concerns already. Positional rank exaggerates small differences in production, so I want to compare preseason projected points per game and actual points per game over the first four weeks. In terms of what players to include, because my old methodology only looked at the top 24/36/48/24 players by preseason ADP, I worry that it missed out on off-the-radar players with strongly early performance, which seems like a group that would favor early-season results. I still need some sort of cutoff (we don't really care whether Trinity Benson finished with 0.9 points per game or 2.5 points per game), but I can evaluate every projected starting quarterback and all skill players projected for at least 5 points per game, which gives us 55 running backs, 79 receivers, and 30 tight ends to start.
I'm going to remove every player who wasn't projected for at least 15 games, which mostly excludes players who were injured or suspended to start the season and quarterbacks who were mired in a competition (think: Trey Lance and Jimmy Garoppolo).
Because I'm using points per game I'm able to include players with smaller sample sizes, but I don't want to be too subject to the whims of outliers, so I'll precommit to removing anyone who didn't play at least two mostly full games in the first sample and at least four in the second. (What do I mean by "mostly full games"? Last year Tua Tagovailoa scored about 16.5 points in Week 1 and was hurt after just four pass attempts in Week 2, missing three weeks. Because of the abbreviated outing, he only averaged 8.4 points per game in the first sample, but in reality, that was over 16 points per full game. Rather than trying to correct this data, I'll simply remove it.)
And because I think correlations are useful but don't tell the entire story, I want to look at two other comparisons. What is the average size of the prediction error (i.e. how big is the difference in points per game between preseason projections or early season production and rest-of-year production)? And what percentage of players finish closer to their preseason projection and what percentage finish closer to their early-season production? The first question should reward getting the outliers right, while the second rewards consistency.
Finally, there are two other questions I'm curious about (though I don't think they're as important as the first three comparisons). For players with especially large splits between preseason projections and early-season production (I'll define this as the top 25% at each position), what percentage finished closer to preseason projections vs. early-season production? Also, did preseason projections or early season production perform better specifically among players who were "league-winners" (I'll define this as the Top 3 qualifying QBs, Top 12 RBs and WRs, and Top 3 TEs in total points over week 5-18)?
Looking at Results
Continue reading this content with a ELITE subscription.
An ELITE subscription is required to access content for Dynasty leagues. If this league is not a Dynasty league, you can edit your leagues here.