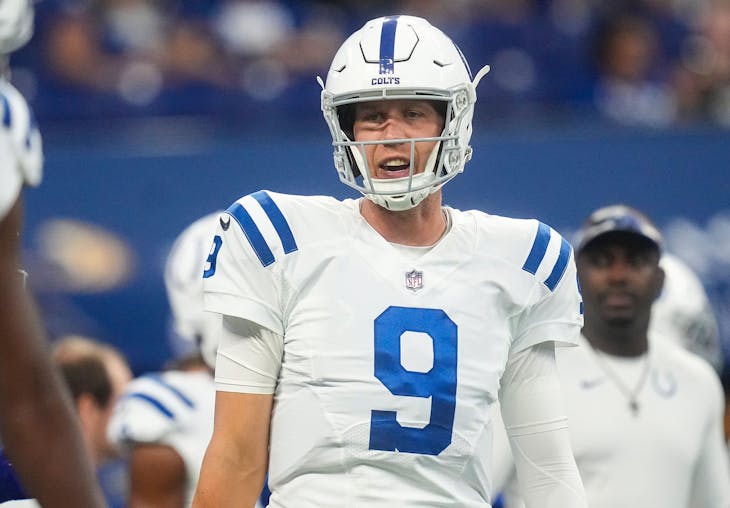
Welcome to Regression Alert, your weekly guide to using regression to predict the future with uncanny accuracy.
For those who are new to the feature, here's the deal: every week, I dive into the topic of regression to the mean. Sometimes I'll explain what it really is, why you hear so much about it, and how you can harness its power for yourself. Sometimes I'll give some practical examples of regression at work.
In weeks where I'm giving practical examples, I will select a metric to focus on. I'll rank all players in the league according to that metric, and separate the top players into Group A and the bottom players into Group B. I will verify that the players in Group A have outscored the players in Group B to that point in the season. And then I will predict that, by the magic of regression, Group B will outscore Group A going forward.
Crucially, I don't get to pick my samples (other than choosing which metric to focus on). If the metric I'm focusing on is touchdown rate, and Christian McCaffrey is one of the high outliers in touchdown rate, then Christian McCaffrey goes into Group A, and may the fantasy gods show mercy on my predictions.
Most importantly, because predictions mean nothing without accountability, I track the results of my predictions over the course of the season and highlight when they prove correct and also when they prove incorrect. Here's a list of my predictions from 2019 and their final results, here's the list from 2018, and here's the list from 2017.
THE SCORECARD
In Week 2, I opened with a primer on what regression to the mean was, how it worked, and how we would use it to our advantage. No specific prediction was made.
In Week 3, I dove into the reasons why yards per carry is almost entirely noise, shared some research to that effect, and predicted that the sample of backs with lots of carries but a poor per-carry average would outrush the sample with fewer carries but more yards per carry.
In Week 4, I talked about how the ability to convert yards into touchdowns was most certainly a skill, but it was a skill that operated within a fairly narrow and clearly-defined range, and any values outside of that range were probably just random noise and therefore due to regress. I predicted that high-yardage, low-touchdown receivers would outscore low-yardage, high-touchdown receivers going forward.
In Week 5, I talked about how historical patterns suggested we had just reached the informational tipping point, the time when performance to this point in the season carried as much predictive power as ADP. In general, I predicted that players whose early performance differed substantially from their ADP would tend to move toward a point between their early performance and their draft position, but no specific prediction was made.
In Week 6, I talked about simple ways to tell whether a statistic was especially likely to regress or not. No specific prediction was made.
In Week 7, I speculated that kickers were people, too, and lamented the fact that I'd never discussed them in this column before. To remedy that, I identified teams that were scoring "too many" field goals relative to touchdowns and "too many" touchdowns relative to field goals and predicted that scoring mix would regress and kickers from the latter teams would outperform kickers from the former going forward.
Statistic for regression | Performance before prediction | Performance since prediction | Weeks remaining |
---|---|---|---|
Yards per Carry | Group A had 3% more rushing yards per game | Group B has 36% more rushing yards per game | Success! |
Yard to Touchdown Ratio | Group A averaged 2% more fantasy points per game | Group B averages 40% more fantasy points per game | Success! |
TD to FG ratio | Group A averaged 20% more points per game | Group B averages 54% more points per game | 3 |
As we bring our yard-to-touchdown ratio prediction to a close, I wanted to recap the changes in production from each group. At the time of the prediction, our Group A receivers were averaging 44.4 yards per game. Since then, they've averaged 45.7. At the time of our prediction, our Group B receivers were averaging 78.3 yards per game; since then they've averaged 64.6. As you can see, there's been a little bit of regression on yards. It hit the high-yardage receivers highest because to some extent their performance to that point was an outlier, which is how they wound up in the high-yardage group. Any receiver with a 200-yard game is more likely to qualify as a "high-yardage receiver", but unlikely to repeat that performance. But despite that regression, the low-yardage players remained low-yardage and the high-yardage players remained high-yardage.
Now let's look at the touchdowns. At the time of our prediction, Group A averaged 0.78 touchdowns per game. Since our prediction, they average just 0.23. At the time of our prediction, Group B averaged 0.17 touchdowns per game; since then they average 0.35. Not only did touchdowns regress much more strongly (dropping by 70% and increasing by 100%, respectively), but they regressed in the direction of yards. The guys who had a lot of yards but few touchdowns started getting more touchdowns. The guys who had a lot of touchdowns but few yards did not start getting a lot of yards; instead, they started getting fewer touchdowns. Touchdowns follow yards, but yards don't follow back.
Four weeks ago, Group A receivers averaged a touchdown for every 57 yards and Group B receivers averaged a touchdown for every 462. Since then, Group A averages a touchdown for every 199 yards and Group B averages a touchdown for every 184. I didn't expect the values to completely flip like that— the ability to convert yards to touchdown is a true skill— but it's no surprise that both groups settled comfortably into that 100-220 yards per touchdown range that we had previously identified as sustainable.
To really put a bow on it: 8 of our 14 "high-touchdown" receivers were held out of the end zone over the last four weeks, (although Cedrick Wilson did throw for a touchdown over that span). Just 3 of our 20 "low-touchdown" receivers (Robby Anderson, Julian Edelman, and Michael Gallup) were held similarly scoreless.
As for our other active prediciton... I really hate to spike the football too hard after a single week because one-week samples are tiny and their results are easy to flip. But four of the five kickers we identified as strong candidates for positive regression set a new season high last week, and the one exception (Greg Zuerlein) can perhaps be forgiven considering the Cowboys under Andy Dalton and Ben DiNucci are not exactly the same as the Cowboys under Dak Prescott. (This is a situation where not being able to manually include or exclude players in my sample is a real bummer.)
Granular Measures
Bill Parcells once said that you are what your record says you are, but of course, you're not. If you have a 2-point lead with five seconds left and the other team is lining up to kick a 50-yard game-winning field goal, whether or not they make the kick has a big impact on what your record says you are but virtually no bearing on what you are. Wins are a very blunt unit of measurement.
In fact, a good rule of thumb when it comes to regression is that the blunter the measure, the more likely regression becomes. Yards and touchdowns are both a decent measure of receiver quality, but touchdowns are much blunter while yards are more granular; accordingly, touchdowns regress much more strongly. Quarterbacks are tasked with avoiding both sacks and interceptions, but sacks are much more common events (to this point, there have been 2.7 times as many sacks as interceptions in the league), so they tend to be a more stable measure of quarterback skill. (Yes, despite what you may have heard, sacks are a quarterback stat.)
Wins are likewise very rare events. In fact, NFL teams average fewer wins per game (0.5) than interceptions per game (0.8). And as a blunt measure, they tend to be worse than a more granular measure like, say, point differential.
Right now four teams are 5-1. The Baltimore Ravens and Green Bay Packers have a point differential of 113. The Tennessee Titans and Seattle Seahawks have a point differential of 66.
Right now six teams are 5-2. The Tampa Bay Buccaneers, Arizona Cardinals, and Los Angeles Rams have a combined point differential of 189. The Chicago Bears, Buffalo Bills, and Cleveland Browns somehow have a combined point differential of -27.
Right now two teams are 4-2. The Colts have a point differential of 42. The Saints have a point differential of 6.
Right now four teams are 1-6. The Atlanta Falcons and Houston Texans have a point differential of -74, while the Jacksonville Jaguars and New York Giants have a point differential of -118.
That's a list of every record that is common to an even number of teams (so I can split them evenly between my two groups), with the best teams (by point differential) separated from the worst. This gives us our two groups to watch.
The Ravens, Packers, Buccaneers, Cardinals, Rams, Colts, Falcons, and Texans have a combined record of 31-22 and a combined point differential of 270 points. This is our Group A. The Titans, Seahawks, Bears, Bills, Browns, Saints, Jaguars, and Giants have an identical 31-22 record, but a point differential of -73. There's our Group B.
Both Group A and Group B have identical winning percentages so far, but Group A is faring much better in the more granular measure of point differential. Since granular measures are much less likely to regress, I would expect Group A to win games at a significantly higher rate going forward. Let's say a 10% edge to make it sporting, roughly equivalent to three extra wins (assuming all games are played).